RESEARCH HIGHLIGHTS
379
NYCU
Number of Academic Papers
Published by Students or Exhibition
Activities Organized by Students
- Update Date:2024-07-16
- Units:Office of International Promotion and Outreach
The solution to Phone Overheating! NYCU Research Team Breaks Through Multi-Core Chip Heat Management, Wins IEEE TVLSI Best Paper Award
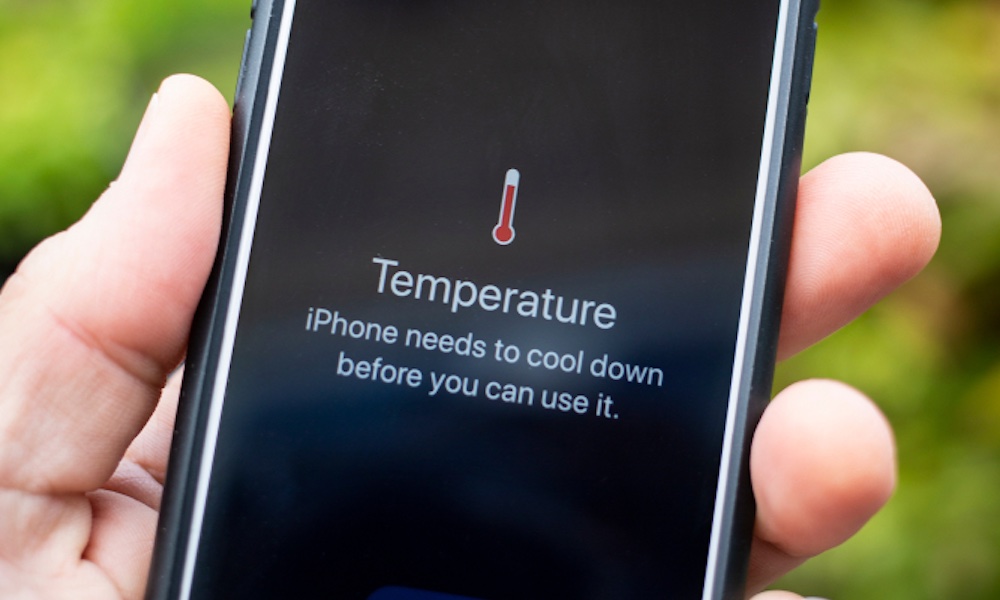
(Photo credit: iDropNews)
Translated by Hsuchuan
Edited by Chance Lai
______
Edited by Chance Lai
______
Overheating phones drag down system performance and affect users’ moods. The Cerebral and Reliable SoC Laboratory (CERES Lab) at National Yang Ming Chiao Tung University (NYCU) has developed a temperature prediction and control technology for multi-core chip networks, which enhances heat dissipation and alleviates overheating issues. This research achievement has been awarded the Best Paper Award by the international journal IEEE TVLSI (IEEE Transactions on Very Large Scale Integration Systems), marking the first time in 30 years that a team from Taiwan has received this honor.
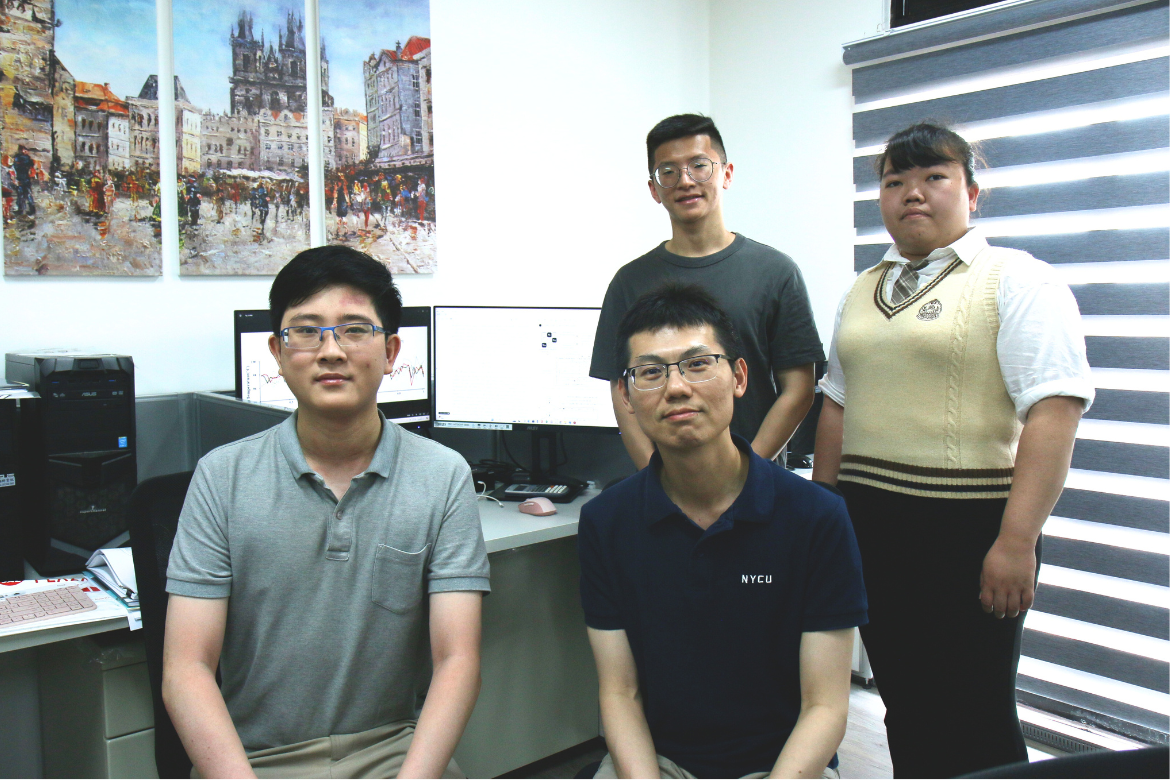
Led by Associate Professor Kun-Chih Chen (front row, right), the CERES Lab research team has made breakthroughs in multi-core chip thermal management technology.
Multi-Core Chips Essential for Computers and Phones, Temperature Management Key to Enhancing Performance
In recent years, multi-core chips have been widely used in computers, smartphones, servers, and other devices. As the number of processor cores increases, the Network on Chip (NoC) connectivity structure has become a widespread technical issue. Additionally, the rise in the clock frequency of computing cores poses significant temperature challenges, significantly affecting chip performance and reliability.
Associate Professor Kun-Chih Chen of the Institute of Electronics led the CERES Lab research team, which included graduate students Yuan-Hao Liao, Cheng-Ting Chen, and Lei-Chi Wang. They proposed a low-cost online learning mechanism for accurate temperature prediction in NoC systems. Using adaptive reinforcement learning technology, they implemented dynamic, proactive temperature management to address the temperature challenges of multi-core chips, significantly enhancing the system’s temperature management performance.
The research team explained that the thermal issues in NoC systems require real-time system temperature monitoring. Dynamic thermal management mechanisms are triggered when the system temperature reaches dangerous levels to prevent overheating. Proactive Dynamic Thermal Management (PDTM) controls the system temperature in advance based on temperature prediction information. Using partial throttling schemes, PDTM reduces performance impact during temperature control, making it more effective than traditional reactive dynamic thermal management.
Multi-Core Chips Essential for Computers and Phones, Temperature Management Key to Enhancing Performance
In recent years, multi-core chips have been widely used in computers, smartphones, servers, and other devices. As the number of processor cores increases, the Network on Chip (NoC) connectivity structure has become a widespread technical issue. Additionally, the rise in the clock frequency of computing cores poses significant temperature challenges, significantly affecting chip performance and reliability.
Associate Professor Kun-Chih Chen of the Institute of Electronics led the CERES Lab research team, which included graduate students Yuan-Hao Liao, Cheng-Ting Chen, and Lei-Chi Wang. They proposed a low-cost online learning mechanism for accurate temperature prediction in NoC systems. Using adaptive reinforcement learning technology, they implemented dynamic, proactive temperature management to address the temperature challenges of multi-core chips, significantly enhancing the system’s temperature management performance.
The research team explained that the thermal issues in NoC systems require real-time system temperature monitoring. Dynamic thermal management mechanisms are triggered when the system temperature reaches dangerous levels to prevent overheating. Proactive Dynamic Thermal Management (PDTM) controls the system temperature in advance based on temperature prediction information. Using partial throttling schemes, PDTM reduces performance impact during temperature control, making it more effective than traditional reactive dynamic thermal management.
Machine Learning Helps NoC Systems Overcome Temperature Prediction Challenges
The temperature behavior of NoC systems varies with different workload distributions, making it difficult to accurately capture physical parameters such as capacitance, resistance, and power during operation, leading to significant temperature prediction errors. In recent years, machine learning prediction methods have dynamically accommodated the hyperplane of physical system behavior. However, machine learning methods depend highly on the quality of training data, resulting in considerable errors in NoC systems.
Associate Professor Kun-Chih Chen stated that the research team’s machine learning-based proactive temperature management employs the least mean squares adaptive filtering theory to optimize the model. This approach dynamically adjusts temperature predictions, enhancing accuracy to cope with varying workloads and temperature changes.
The method introduces adaptive reinforcement learning, using real-time feedback on current temperature, predicted temperature, and system throughput to dynamically adjust throttling ratios, achieving optimal thermal management while maximizing system performance. The research results show that, compared to traditional methods, the proposed adaptive reinforcement learning method significantly reduces temperature prediction errors and improves system performance.
This innovative research achievement was selected for the 2024 IEEE TVLSI Best Paper Award, representing the highest recognition for the research team and highlighting NYCU’s exceptional research contributions and advanced technology development capabilities.
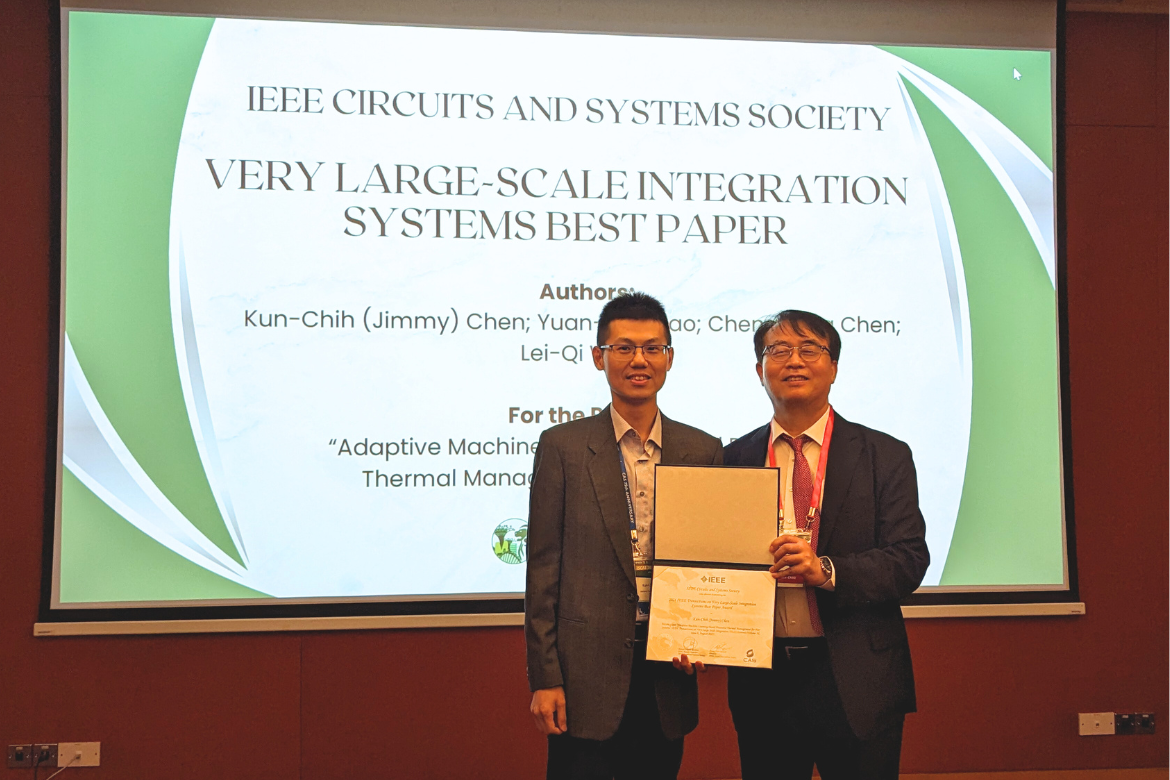
Paper Title: Adaptive Machine Learning-Based Proactive Thermal Management for NoC Systems
Related Image(s):